FLUX-Controlnet-Inpainting: Transforming Image Restoration with AI Precision
Dive into the transformative world of FLUX-Controlnet-Inpainting, where advanced AI meets image restoration! Discover how this revolutionary tool integrates deep learning techniques for stunning results and learn step-by-step how to harness its power for your creative projects.
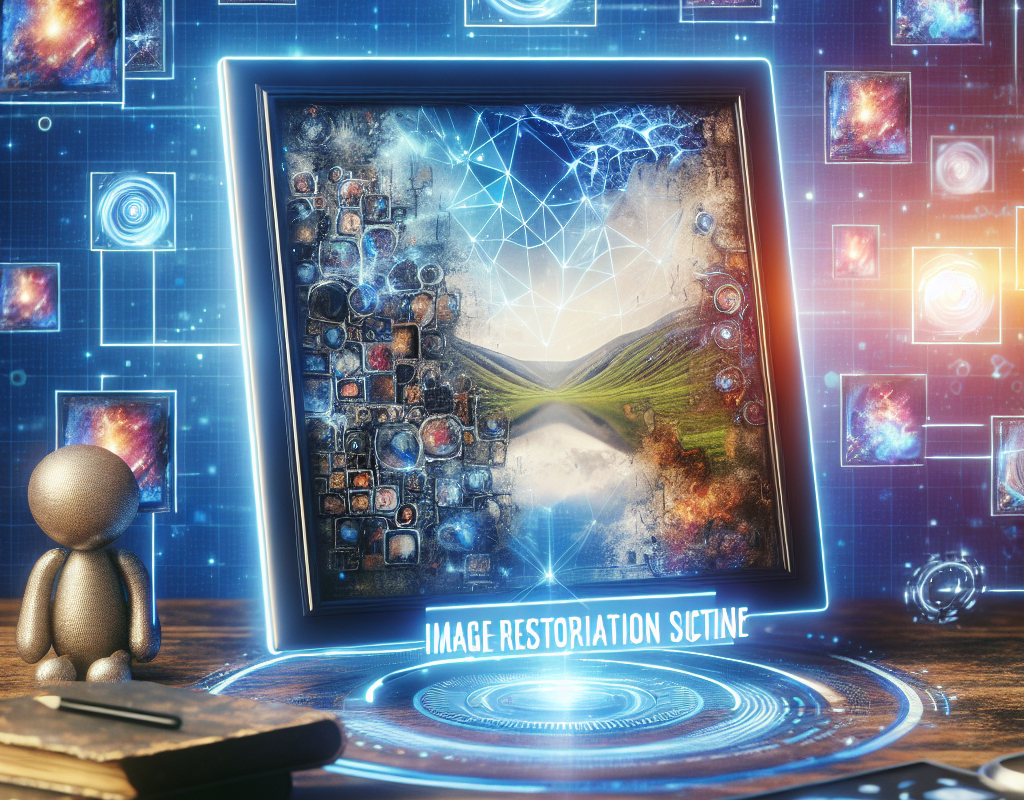
FLUX-Controlnet-Inpainting: Revolutionizing Image Restoration
Table of Contents
Introduction
In the ever-evolving landscape of artificial intelligence, image restoration has become a crucial aspect of digital media. The recent launch of FLUX-Controlnet-Inpainting by Alimama Creative has significantly advanced the field of image repair, offering a powerful tool that leverages the strengths of ControlNet and the FLUX.1-dev model. This innovative solution is designed to accurately repair images by utilizing information such as edges, line drawings, and depth maps, generating content in specified areas that harmoniously blends with the surrounding environment.
What is FLUX-Controlnet-Inpainting?
FLUX-Controlnet-Inpainting is an image restoration tool based on the FLUX.1-dev model and ControlNet architecture. It is trained on a large dataset of 12 million images from the laion2B dataset and other internal sources, all at a resolution of 768x768. This high-resolution training ensures that the model performs best at this size, with other resolutions yielding suboptimal results.
Key Features
High-Quality Image Generation
The FLUX-Controlnet-Inpainting model is capable of performing high-quality inpainting on images. By using a control image as a guide, the model can intelligently fill in missing regions of an input image, producing natural-looking results.
Integration with ComfyUI
Recently, FLUX's Inpainting ControlNet has achieved a breakthrough in inference capabilities through integration with ComfyUI. This means users can now perform complex image restoration tasks in an intuitive interface, enjoying a smooth workflow.
Optimized Inference Speed
When using the t5xxl-FP16 and flux1-dev-fp8 models for 28-step inference, approximately 27GB of GPU memory is required. However, the inference speed remains satisfactory: at cfg=3.5
, it takes only 27 seconds; if cfg
is reduced to 1, it can be shortened to 15 seconds. For users seeking faster speeds, Hyper-FLUX-lora offers an excellent option, significantly enhancing inference efficiency.
Parameter Adjustments
To achieve optimal results, users can fine-tune key parameters such as control-strength, control-end-percent, and cfg. For example, setting control-strength to 0.9, control-end-percent to 1.0, and cfg to 3.5 often achieves an ideal balance.
How to Use
Using FLUX-Controlnet-Inpainting is straightforward and can be integrated into various workflows. Here’s a step-by-step guide to get you started:
Step 1: Installation
First, you need to install ComfyUI and follow the basic Flux installation workflow if you haven’t yet. From the Comfy Manager, click on "Custom nodes Manager" and install "ComfyUI essentials" by Cubiq.
Step 2: Downloading the Model
Download the FLUX inpainting model (safetensors) weight from Alimama creative's Hugging Face repository. Save it inside the "ComfyUI/models/controlnet" folder. You can rename it to anything relative like "Alimama-Flux-controlnet-inpainting.safetensors" for a well-structured workflow.
Step 3: Setting Up the Workflow
- Download the Workflow: Download the workflow from Alimama Creative Hugging Face. This uses the native Flux Dev workflow.
- Drag and Drop: Drag and drop the workflow into ComfyUI. If you get red-colored error nodes, then from Manager click "Install missing custom nodes." Restart and refresh your ComfyUI to take effect.
- Load Model and Image: Choose "Flux.1 Dev" as the model weight from "Load diffusion model" node. Load ControlNet inpainting model which you downloaded from "Load ControlNet model" node. Load your target image in "Load image" node. Right-click on the image and select option "Open in Masked Editor." Mask the area where you want to influence it and click "Save to node".
- Configure Settings: Add descriptive positive prompts in "Clip text encode" node. Configure settings by adjusting parameters like control-strength, control-end-percent, and cfg. A great starting point is setting control-strength = 0.9, control-end-percent = 1.0, and cfg = 3.5.
- Initiate Inpainting: At last, click the "Queue" button to initiate your image generation.
Pricing
The FLUX-Controlnet-Inpainting model is currently available under the FLUX.1 dev Non-Commercial License. This means that while the model is free to use for non-commercial purposes, it is not open-source and comes with specific usage restrictions. For commercial use, you would need to contact Alimama Creative for licensing details.
FAQ
Q: What is the recommended resolution for using FLUX-Controlnet-Inpainting?
A: The recommended resolution for using FLUX-Controlnet-Inpainting is 768x768. This resolution ensures optimal performance and high-quality results.
Q: How much GPU memory is required for inference?
A: When using the t5xxl-FP16 and flux1-dev-fp8 models for 28-step inference, approximately 27GB of GPU memory is required.
Q: Can I adjust the parameters to achieve better results?
A: Yes, you can adjust parameters like control-strength, control-end-percent, and cfg to achieve better results. For example, setting control-strength to 0.9, control-end-percent to 1.0, and cfg to 3.5 often achieves an ideal balance.
Q: Is the current version an alpha version?
A: Yes, the current version of FLUX-Controlnet-Inpainting is an alpha version. The development team promises more powerful updates in the future.
Q: What kind of images can I restore using this tool?
A: FLUX-Controlnet-Inpainting can restore a wide range of images, including photos, graphics, and illustrations, making it a versatile tool for both artists and professionals.
By leveraging the strengths of ControlNet and the FLUX.1-dev model, FLUX-Controlnet-Inpainting is poised to revolutionize the field of image restoration. Its high-quality image generation capabilities, optimized inference speed, and intuitive interface make it an essential tool for any professional or enthusiast looking to enhance their image repair tasks. As the technology continues to evolve, we can expect even more astonishing breakthroughs in the future of image restoration.